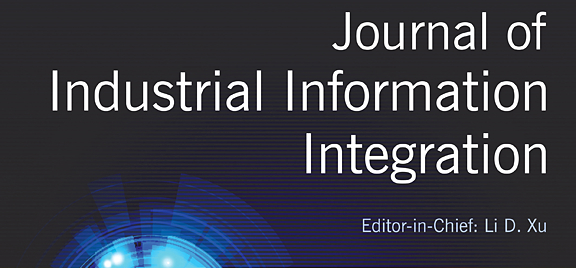
A recent study from SocketSense, in collaboration with other researchers, sheds light on enhancing the accuracy of flexible wearable sensors in prosthetic limbs.
Stockholm, August 21st 2023 – Researchers from SocketSense have recently collaboratively explored a refined approach to detect anomalies in data obtained from flexible wearable sensors in limb prostheses. Their findings are elaborated upon in the journal article titled “Using a VAE-SOM Architecture for Anomaly Detection of Flexible Wearable Sensors in Limb Prosthesis.” This paper was a joint effort from Fudan University(Zikai Zhu, Jiayu Huang, Zhuo Zou, Lirong Zheng), KTH Royal Institute of Technology(Peng Su, Suranjan Ottikkutti, Kaveh Nazem Tahmasebi, DeJiu Chen) and Linköping University(Sean Zhong).
Wearable sensor electronics have seen consistent advancements, leading to their integration into various intelligent healthcare devices. One application that stands to benefit is limb prostheses. Such sensors offer potential in monitoring and assessing the functioning of prosthetic limbs in diverse environments. However, the data can sometimes present inconsistencies and errors.
To address this, the research team investigated an approach that combines several computational models. The method involves the Variational AutoEncoder (VAE) to first encode the multi-variant time-series data into latent states. Subsequently, the Self-Organizing Map (SOM) technique creates discrete representations of these states.
To further the accuracy of anomaly detection, the team considered adaptive Markov chains, which help quantify state transitions and detect any potential inconsistencies.
Key insights from the research include:
- The potential of the VAE-SOM hybrid model to transition continuous data into discrete states, aiding in clearer data interpretation.
- The incorporation of adaptive Markov chains to understand state transitions, offering a more nuanced view of prosthetic operations.
When compared to several benchmark methods, the proposed architecture showed promising results. It was tested on two public datasets, providing noteworthy F1 scores. A look into its run-time performance also highlighted its efficiency in terms of computational demands.
This research from our EU project represents a step forward in the realm of prosthetics. By potentially improving anomaly detection, it offers a way to refine the performance of prosthetic limbs equipped with these sensors.
For a deeper insight into the methodology and its far-reaching implications, the full journal article is available here for interested readers.